Modelling When Customers Will Purchase: How to Predict Lifetime Value
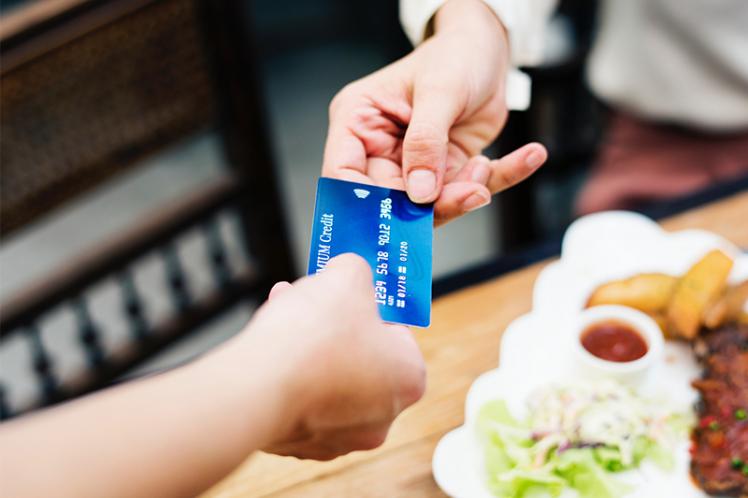
Incorporate survival analysis into your strategy to help predict consumer behaviour
Typically, lifetime value (LTV) is a calculation that uses descriptive or historical data. This calculation tends to make assumptions for the future, yet usually provides little insight into why a customer might be lower-valued, or how to make a customer higher-valued. Essentially, descriptive techniques offer no insights into predicting, incenting, or changing customer behaviour.
However, using predictive techniques such as survival analysis allows an indication of what causes purchases to happen sooner (or later) to be discovered. This means marketers gain insight into how to increase LTV, making the predictive modelling more strategically lucrative.
LTV is a summary calculation accumulated by past net revenue, which is then applied to each customer. This is typically used for marketers to find their ‘high value’ customers (based on past purchases), so they know who to target their communications and marketing efforts to. This is a great starting point – but doesn’t contribute any information on why.
An Introduction to Survival Modelling
Survival analysis is about modelling the time leading up to an event, be that a response, purchase, or visit. There are a couple of important characteristics of survival analysis:
- The dependent variable is time-until-an-event, so time must be built into the analysis
- Survival analysis accounts for observations that are censored. A censored observation is either an observation that has not had the event or an observation that was lost to the study with no knowledge of having the event or not
In marketing, it’s common for the event to be a purchase. This means a database of customers can be scored with ‘time-until-purchase’. That is far more actionable than, from logistic regression, the probability of purchase.
By using survival analysis, marketers can see which independent variables, such as lowering price, tend to decrease time-until-purchase. This decreasing time-until-purchase often increases LTV. While survival analysis can predict the next time until purchase, the strategic value of survival analysis is in using the independent variables to change the timing of purchases.
Essentially, descriptive analysis shows what happened; predictive analysis gives a glimpse of what might change the future.
If a customer purchases sooner, there is an increased chance of adding purchases. But even if the number of purchases is not increased, getting revenue sooner will add financial value.
A business case can be created by showing the trade-off in giving up margin but obtaining revenue faster. This means a strategy can revolve around maximization of cost, balanced against customer value.
The idea is to model the next time-until-purchase (the baseline) and see how to improve that. How is this carried out? A behaviorally-based method would be to segment the customers (based on behaviour) and apply a survival model to each segment and score each individual customer. Behaviour typically means purchasing (amount, timing, share of products) metrics from marketing communications (open and click rate, direct mail coupons) and responses.
Example Case Study
The table below shows two customers from two different behavioural segments. Customer X purchases every 88 days with an annual revenue of 43,958, costs of 7,296, for a net revenue of 36,662. If the second year were the same, the year 1 (discounted at 9%) is NPV of 33,635, while year 2 (discounted at 9% for two years) becomes 30,857 for a total LTV of 64,492.
Customer Y shows similar calculations for LTV of 87,898.
The above (using descriptive analysis) would have marketers targeting customer Y with > 23,000 value over customer X. But do we know anything about WHY customer X is valued lower? Is there anything that can be done to make customer X higher valued?
Applying a survival model to each segment outputs independent variables and shows their effect on the dependent variable. In this case, the dependent variable is (average) time-until-purchase. Say the independent variables (which defined the behavioural segments) are things like price discounts, product bundling, seasonal messaging, adding additional direct mail catalogues, offering online exclusives, etc., then the segmentation should separate customers based on behaviour, and the survival models should show how different levels of independent variables drive different strategies.
The next table shows the results of survival modelling on the two different customers that come from different segments. The independent variables are price discounts at 10%, product bundling, etc. ‘TTE’ represents time-until-event and demonstrates the effect on time-until-purchase, based on changing one of the independent variables.
For customer X, giving a price discount of 10%, on average, decreases their time until purchase by 14 days. Giving Y a 10% discount, however, decreases their time until purchase by only 2 days. This means customer X is far more sensitive to price then customer Y- which would not be known by descriptive analysis alone. Likewise, offering X more direct mail catalogues push out their TTE but pulls in Y by 2 days. Note also that very little of the marketing tactics affect Y. We are already exceeding almost all we can from Y, with marketing efforts having little impact on the TTE. However, with X, there are several things that can be done to encourage their purchase. All of which would not be known without conducting survival modeling on each behavioral segment.
The table below shows new LTV calculations on customer X after using survival modelling results. We decreased TTE by 24 days, using a combination of discounts, bundling and online exclusives. Note that the LTV for customer X (after using predictive analysis) is now greater than Y.
What survival analysis offers, therefore, in addition to marketing strategy levers, is a financial optimal scenario, particularly in terms of costs to market. That is, customer X responds to a discount. This makes it possible to calculate and test what is the (just) needed threshold of discounts to bring in a purchase by so many days, with the estimated level of revenue. This ends up being a cost/benefit analysis for marketers to think about their strategy, which is an advantage of predictive analysis; providing marketers with strategic options.
Conclusion: The Advantages of Survival Analysis
In terms of lifetime value, using survival analysis offers the following advantages:
1. It's statistical, rather than merely mathematical. This means it will not suffer as much from any recent change in a customer's buying behaviour. For example, if a customer severely decreases their purchases in recent time periods, predictive analytics will catch and adjust to this much quicker than descriptive analysis (which tends to rely on historical data). The converse is also true.
2. It's predictive. LTV can be estimated by several cause-and-effect (independent) variables. These variables (especially marketing levers like price, discounts, marketing communications, product bundling, etc) provide insight into how to change and increase LTV.
3. Financial business cases can be made. If it costs 5% more in discounts to increase a customer's LTV, the model can show the increase offers about a 2% increase in LTV.
4. It helps generate a marketing strategy, rather than simply targeting high-value customers.